
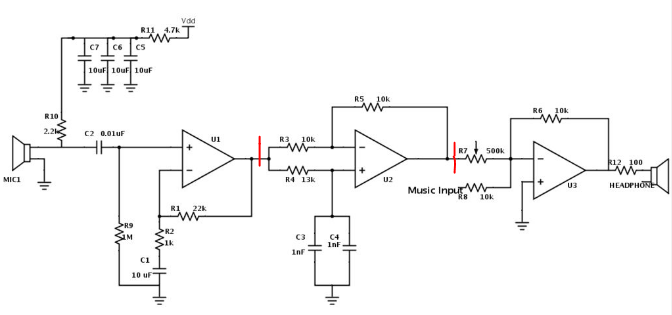
The unexpected poor performance by DCCRN and Conv-TasNet could be due to the limited number of epochs all models are trained on, as well as inherent shortcomings in their architectures. Interestingly, NSNet, which performed the best among the baselines on the synthetic test set for most metrics, has the simplest architecture amongst the baselines and also does not use pooling. This demonstrates the ability of cD3Net architecture to learn useful intermediate features in a very parameter-efficient manner. In both the synthetic and real test sets, cD3Net models of any configuration consistently outperformed the three baseline models across most metrics, despite only having about a tenth of the parameters of the smallest baseline model. Metric values within 0.01 of the respective top scores are considered practically equivalent. Legends for MOS scores: NE – singletalk nearend MOS FE – singletalk farend Echo DMOS DT/E – doubletalk Echo DMOS DT/O – doubletalk Other DMOS. Table 1: Evaluation results on the synthetic test set and real test set. As per the convention in the ICASSP and Interspeech AEC Challenges, we report MOS for the nearend singletalk scenario, Echo DMOS for the farend singletalk scenario, and both Echo and Other DMOS for the doubletalk scenario.Ĭonv-TasNet Both DMOS are rated on a 1-to- 5 scale, with a higher score reflecting a better audio quality. The Echo DMOS score captures degradation due to farend echo while the Other DMOS captures degradation due to any other sources. The AECMOS provides two degradation MOS (DMOS) scores. On the other hand, neural modules, once trained, operate on a fixed set of weights and provide consistent enhancement without the need for an initial convergence period.įor the blind real test set, we evaluate the model using objective proxies of mean opinion score (MOS) via the DNSMOS system and degradation MOS via the AECMOS system. In particular, modules such as adaptive filters and delay compensators require a ‘warm-up’ period for convergence and can be susceptible to changes in the acoustic environments, especially in a doubletalk scenario. Moreover, neural networks are often capable of performing both linear and nonlinear filtering, rendering the linear DSP modules somewhat redundant and inefficient. , with the differences mainly lying in the sources of degradation. Both speech enhancement tasks share a common goal of retrieving the clean speech term s Given that AEC, RES, and DNS share more similarities than differences, a more unifying formulation naturally follows.
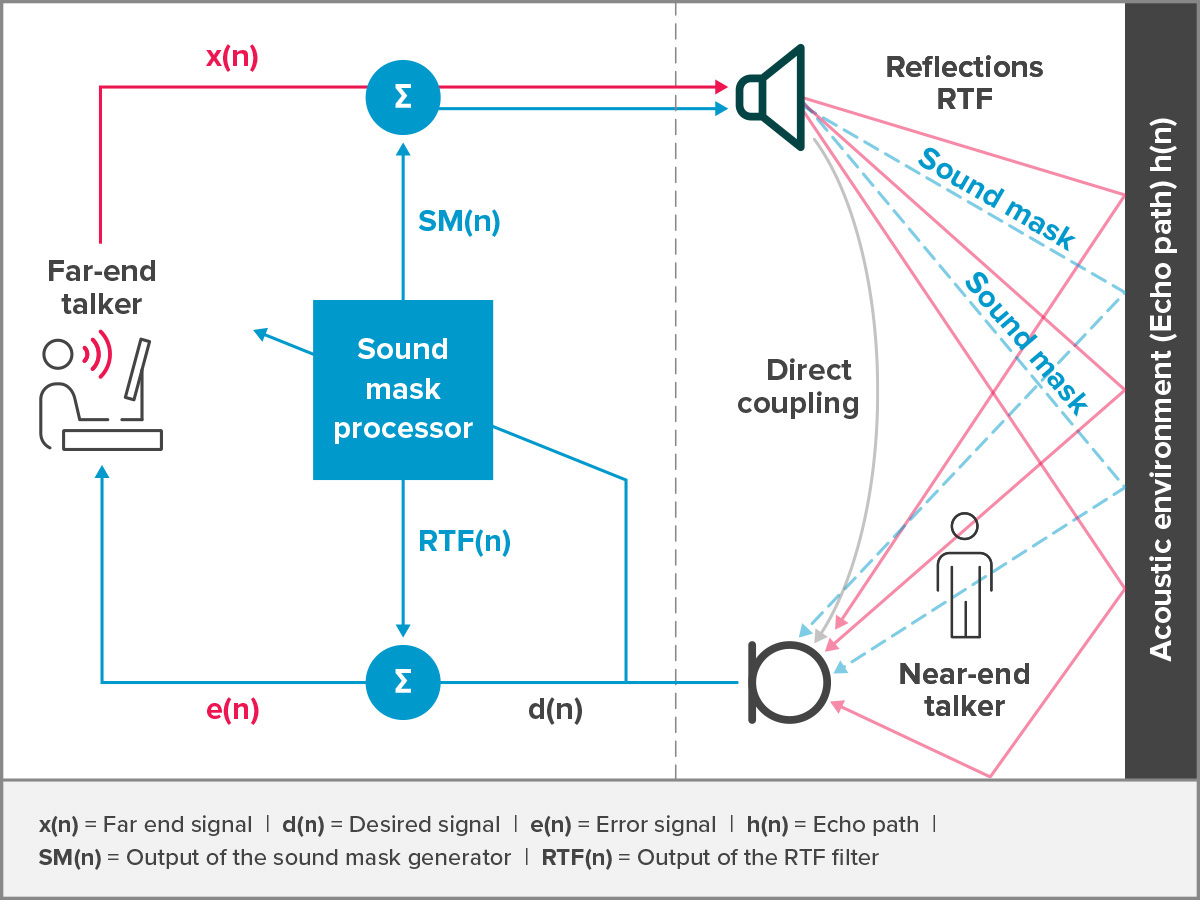
In a broad sense, the residual echoes can be seen as a noise source, thus a few works have adapted DNS models as the RES module for AEC systems. Neural RES is highly related to the task of deep noise suppression (DNS), the latter focusing on noise removal rather than echo cancellation. Evaluation on both syntheticĪnd real test sets demonstrated promising results across multiple energy-based Suppression with simultaneous speech enhancement. We also propose a dual-mask technique for joint echo and noise The architecture utilized the multi-resolution nature of the D3Netīuilding blocks to eliminate the need for pooling, allowing the network toĮxtract features using large receptive fields without any loss of output (D3Net) building block, resulting in a very small network of only 354K Pseudocomplex extension based on the densely-connected multidilated DenseNet The building block of the proposed model is a

In this paper, we exploit the offset-compensating ability ofĬomplex time-frequency masks and propose an end-to-end complex-valued neural Require convergence and remain susceptible to changes in acoustic environments,īut this two-stage framework also often introduces unnecessary delays to theĪEC system when neural modules are already capable of both linear and nonlinearĮcho suppression. However, not only do adaptive filtering modules

Many recent acoustic echo cancellation (AEC) systems rely on a separateĪdaptive filtering module for linear echo suppression and a neural module for Echo and noise suppression is an integral part of a full-duplex communication
